The first step towards checking the pulse of your business is asking existing customers the right questions.
Most enterprises get this right.
It is what they have done with all this data that has made all the difference.
Qualitative answers are as rich in insight as they hard to mine manually. Fortunately, the latest advances in artificial intelligence have made it possible for almost anyone to quickly analyze large and open-ended responses. One such tool is the Google Sheets add-on by Komprehend. It lets you perform survey analysis in your own spreadsheet and is very straightforward to apply.
These tools can be used for a multi-level exploration of information that could otherwise have been easily overlooked.
Level 1 - Scouting for Sentiment

There is a need to distinguish between positive and negative feedback while performing survey analysis. Negative feedback is a goldmine of potential product enhancements, and positive feedback tells you where you are adding the most value to your customers. Using the add-on makes it super easy to use AI and find out the underlying sentiment behind thousands of text pieces in one go. Check the demo of Sentiment Analysis here.
Level 2 - Understanding emotion
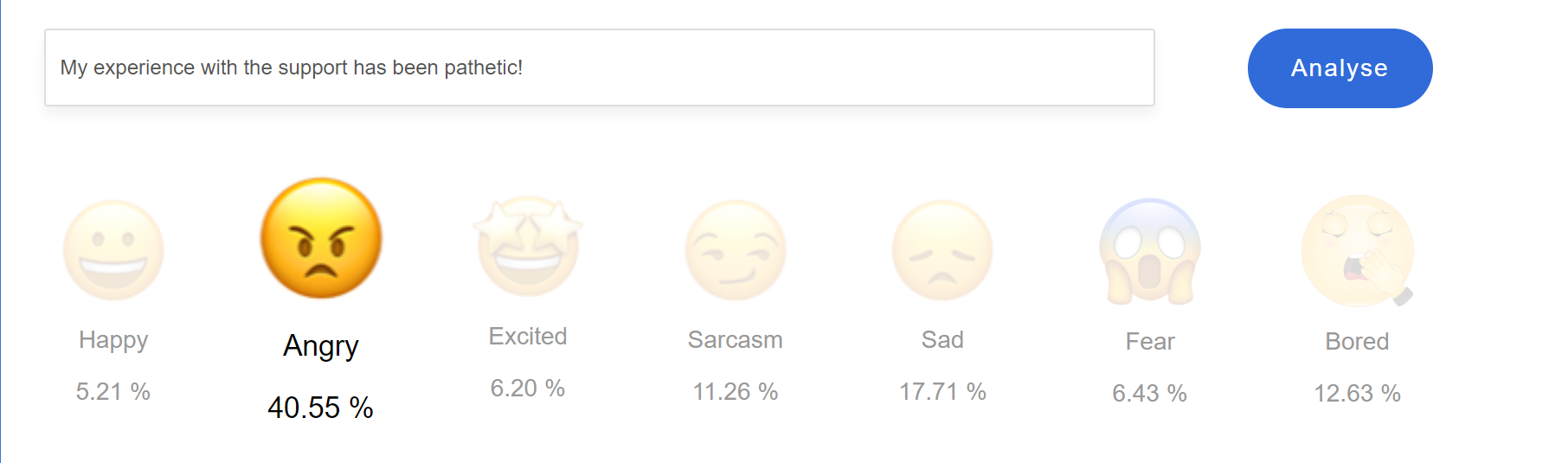
Emotions go one step further than sentiments. Emotion analysis reveals if a customer’s response is - happy, sad, sarcastic, angry, bored, fearful or excited. Imagine the fury of a customer whose feedback is angry with a score of 0.95. Prioritizing user’s complaints using Emotion Analysis score can help you in taking actions quickly. Check the demo of emotion analysis here.
Level 3 - Finding keywords
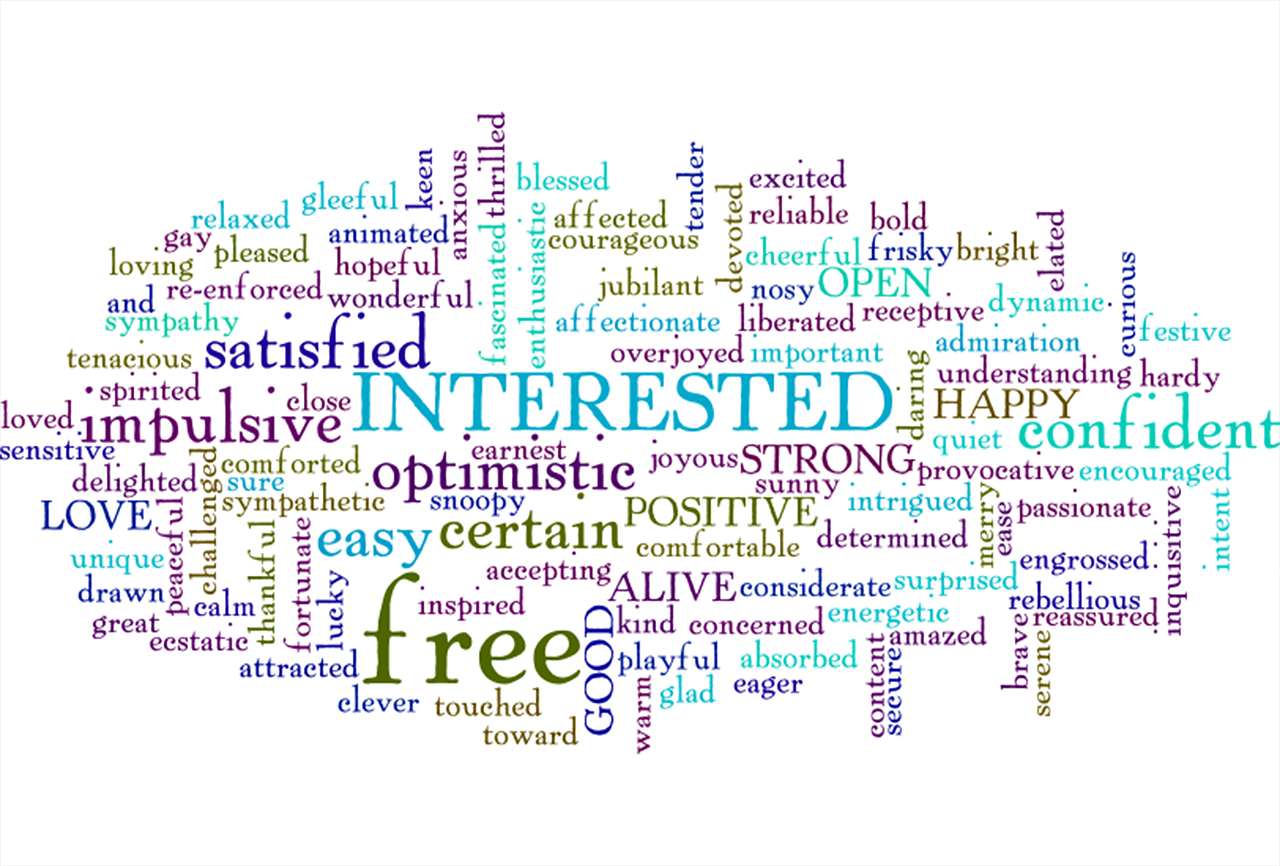
Keyword Generator can be used when you need to generate tags and meta-data from any text corpus for retrieving important information quickly. Keyphrases are similar to keywords and should be used when you want to analyze text corpus to find major topics people were talking about in the corpus.
For example: consider a text like “This restaurant is always congested.”
Keyword API will give “restaurant” as a keyword which can be used to tag this feedback while Key Phrase API will give “always congested” as a phrase. For an educational content provider, he already is aware of the fact that course will appear frequently in user feedback but a phrase like “too long” is important for him to know the real feedback.
Level 4 - Classifying data into best fitting topics
In a classification scenario, we run a pattern discovery algorithm over a small set of labeled training data to compute text patterns that are highly correlated with the occurrence of a specific label (i.e. if the pattern occurs, then – with high probability – so does the label). The classifier identifies relationships between the words and stores them for analyzing unseen future documents.
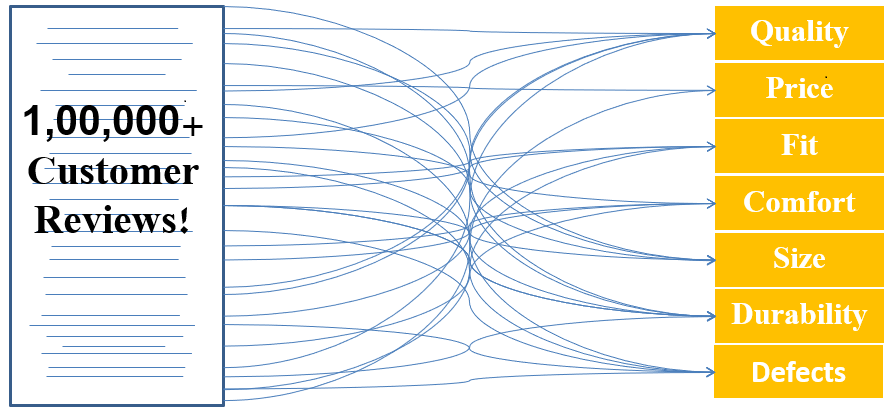
Consider the task of classifying user-feedback emails sent to a large company into emails expressing positive and negative sentiment. In this context, a frequent text pattern that has a high correlation to the negative label might be “I will switch to XYZCorp”, where XYZCorp is the name of a competitor. Once the classifier has learned this, it will be able to classify other new documents into labels, just like a human would.
More specific to customer surveys, let’s consider the following example.
An international sportswear brand received 10,000 reviews on its newly launched line of t-shirts. Here’s a summary of how Komprehend used its custom classifier to delve into this data.
Step 1 - We trained our algorithm on these reviews. The model learns the sentence structure and context of the reviews.
Step 2 - We identified 7 broad topics of concern that emerge from customer reviews - fitting, price, quality, comfort, size, durability, defects. Each topic was annotated with subtopics.
Step 3 - The Custom Classifier automatically analyzed each review and categorized them into relevant topics.
By analyzing conversations for both sentiment and the topics driving that sentiment, a retail bank might discover, that of customers’ criticisms, queue length and waiting times feature uppermost.
A fast-food chain might be interested to know that relative to their closest competitor, many consider their portion size too small, though their friendly customer service is a plus.
At Komprehend, we are making it very easy for our users to use Machine Learning based text classification solutions with no background in data science.
You can either use one of our off-the-shelf text classification solutions like Sentiment Analysis and Emotion Analysis or build your own classifier using Custom Classifier API. All the APIs are available in Google Sheets plugins and Google Sheets add-on to do text mining from the comforts of your spreadsheets.
For Enterprises, text classification models can be licensed for on-premise or private cloud deployment to ensure low latency and compliance with privacy laws.
You can also explore more of our text classification solutions here.
You can read here about applications and use cases of text classification.
We hope you liked the article. Please Sign Up for a free Komprehend account to start your AI journey. You can also check demo’s of Komprehend AI APIs here.